Author: Renoux C
Description
While several time-related biases have been described in pharmacoepidemiologic studies,32 immortal time bias is the most common of these biases in studies of drug effectiveness and safety, especially in the context of COVID-19.16 Latency considerations are also relevant when evaluating vaccines against COVID-19.
The study of drug effectiveness and safety presents unique challenges that stem from the time-varying nature of drug exposure. Moreover, the use of previously collected data enables design flexibility that may, if some elements of the design are not adequately implemented, introduce time-related biases. Properly defining and analyzing treatment status over follow-up time is important. Indeed, inappropriate accounting and classification of exposure over time can introduce immortal time bias.33–35 Immortal time corresponds to a period of follow-up during which, by design, the outcome of interest (death or another outcome) cannot occur. In cohort studies, immortal time is typically introduced when exposure status is defined based on a treatment administered at some point during follow-up. The time period between disease diagnosis or cohort entry and the first treatment is “immortal” because the patient has to survive or be outcome-free (owing to censoring of events in the analysis) to be classified as treated. Immortal time bias is then introduced when this immortal period between diagnosis or cohort entry and the first treatment is either misclassified as exposed or excluded from the analysis rather than correctly accounted for as unexposed. Similarly, requiring a minimum number of prescriptions or duration of treatment for the definition of exposure but considering patients as exposed from the first treatment introduces immortal time bias. Given that individuals move in and out of health systems or insurance plans, it can also be tempting to require patients to have a certain amount of enrollment in follow-up (e.g., one year), which also may create immortal time.
What does it look like in practice?
Several recent cohort studies assessing the effectiveness of various drugs in patients hospitalized with COVID-19 defined exposure on the basis of treatment received at any time during follow-up or within a limited time period (such as the first 48 hours of hospitalization) in their primary analysis. 23,26,36–39 However, patients were considered exposed as of the date of admission (cohort entry), thereby introducing immortal time bias (Figure 3.2a). The time period between cohort entry (date of hospitalization) and the first prescription or exposure for the treated patients corresponds to immortal time, as patients must survive or be event-free (i.e., not censored) to receive their first treatment. This immortal person-time should be classified as unexposed until the first exposure (prescription), at which point the patients are classified as exposed. Similarly, a cohort study where only patients with a minimum of 3 days of treatment were included in the analysis but cohort entry was defined as the first day of treatment also introduces immortal time bias.40
In other cohort studies of patients hospitalized with COVID-19, cohort entry was defined as the date of the first treatment during the hospitalization for treated patients and as the date of hospitalization for those not treated, also introducing immortal time bias (Figure 3.2b).41 In this situation, the immortal time period is excluded rather than misclassified.
Why is it a problem?
Immortal time bias is particularly problematic in cohort studies of drug effects because it tends to systematically bias the results downward in favor of the treatment under study. As such, a treatment may appear protective when it is ineffective, or neutral when it is actually harmful.33–35 The latter is illustrated in a study evaluating the effectiveness of hydroxychloroquine and azithromycin in patients with COVID-19, where both the primary analysis with misclassified immortal time and a sensitivity analysis correctly classifying exposed and unexposed person-time, thereby preventing immortal time bias, were conducted. In the primary analysis with immortal time bias, the hazard ratio was 1.35 (95% CI 0.76-2.40) while in the correct sensitivity analysis, the treatment was associated with an increased rate of death (HR 1.83; 95% CI 1.02, 3.28).39 The magnitude of the bias and its impact on the results depends on the number of patients exposed in the cohort, the amount of immortal time misclassified or excluded, the duration of follow-up, and the number of events.33–35
How to handle it
Immortal time bias, as described above, is introduced by the investigator at the design or analysis stage and can thus be easily avoided. A simple first approach at the design phase is to define exposure at the date of cohort entry, in a cohort of patients defined by a first diagnosis or hospitalization for COVID-19. Exposure could also be defined based on treatment initiation during a specific time period, such as in the first 48 or 72 hours of hospitalization, with start of follow-up accordingly moved to 48 or 72 hours after the date of hospitalization. A second approach is to use a time-varying exposure definition at the analytic stage, with each day of follow-up classified as either exposed or not exposed to the drug of interest. Finally, a design approach aimed at emulating a target trial (see Chapter 2) in this setting can be used, such as the prevalent new-user cohort design or the creation of a series of sequential cohorts at predetermined time intervals.42–44
Latency considerations
When evaluating effectiveness of vaccines against COVID-19, latency may be considered in the exposure definition. The latency time period takes into account the biologically relevant timing of exposure. Thus, for a vaccine fully effective >14 days after being injected, patients may be considered unexposed for the first 14 days following vaccine receipt and exposed thereafter. This latency period must be applied to all exposed patients and not only to those with the event of interest during follow-up. The same latency considerations apply when studying the effectiveness of vaccines requiring 2 doses, where patients move from unexposed time period to partially exposed and fully exposed during follow-up. These latency considerations may not apply to all outcomes, and may be irrelevant when studying safety,45 for example.
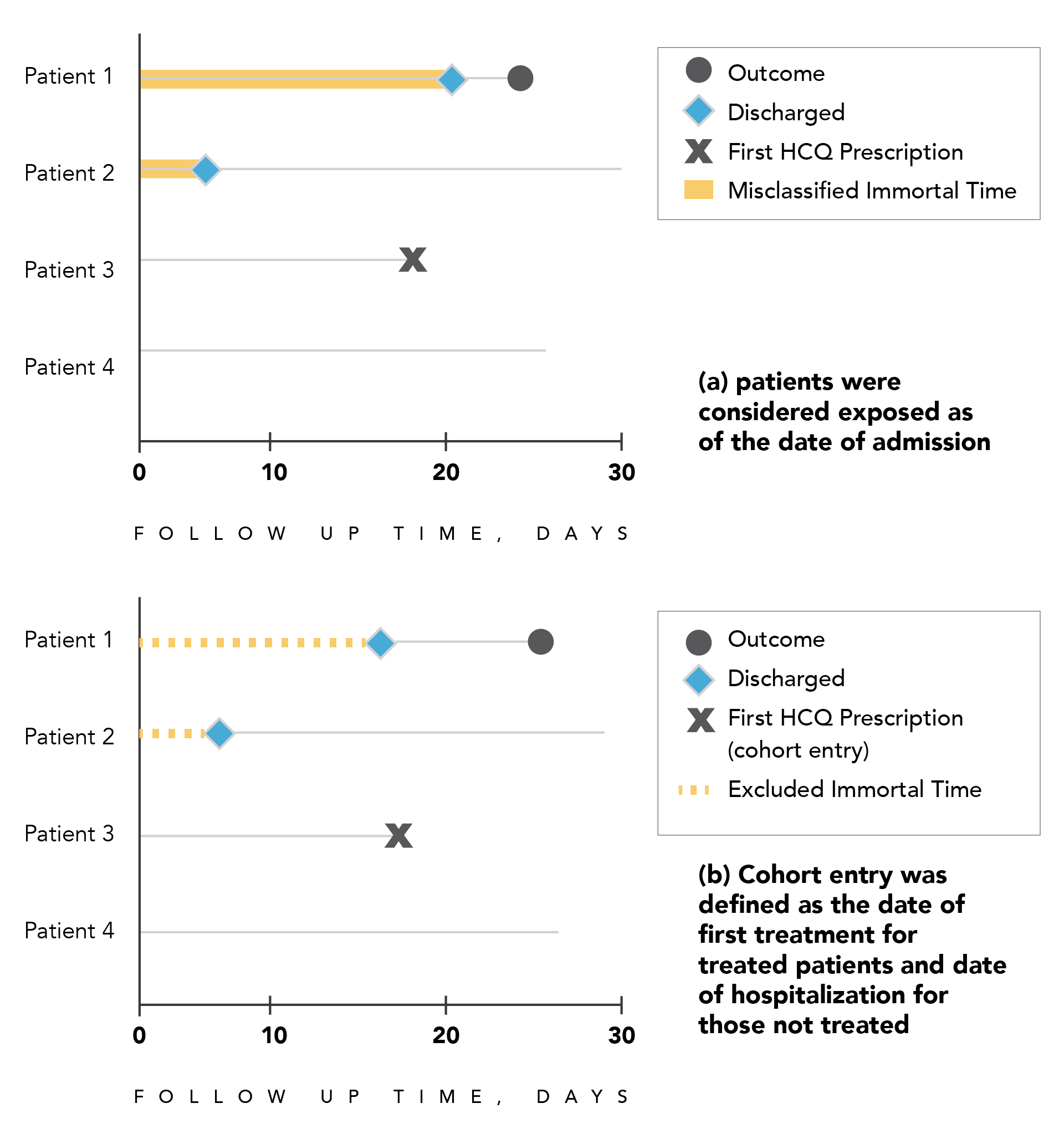
Figure 3.2. Illustration of immortal time bias in a hypothetical cohort of patients hospitalized with COVID-19
Panel A. Patients 1 and 2 receive a first prescription of hydroxychloroquine at some point during hospitalization, but are considered exposed from the date of hospital admission (cohort entry), thereby introducing immortal time bias caused by exposure misclassification (red line).
Panel B. Cohort entry for patients 1 and 2 is defined by the first drug prescription and by the date of hospital admission for the unexposed, thereby introducing immortal time bias caused by excluded unexposed person-time (red dashed line) that should be accounted for in the unexposed group. Abbreviation: HCQ = hydroxychloroquine